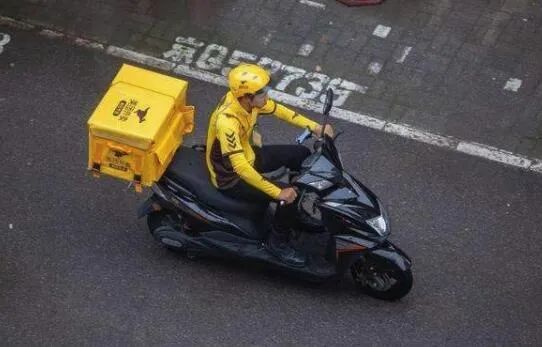
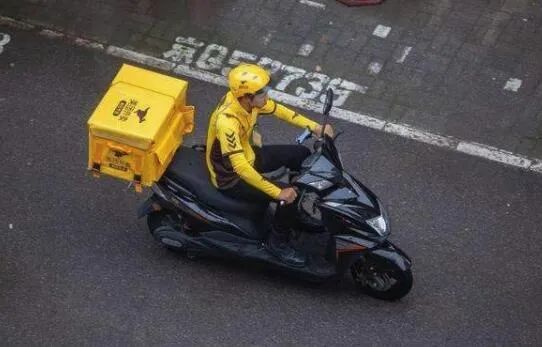
Who makes takeaway riders race against time? What is the logic of the intelligent distribution system?
Recently, a report on the working conditions of delivery riders in People magazine has attracted widespread attention, showing how delivery riders are forced to race against time under the control of ever-accelerating algorithms.
What is the logic behind the food delivery intelligent distribution system? Is it an algorithm that forces takeaway riders to speed at high speeds at the risk of speeding? What is the relationship between the algorithmic world and humans?
In order to answer these questions, the reporter interviewed Wang Zizhuo, co-founder of Shanshu Technology, an artificial intelligence decision-making company, and associate professor of the School of Data Science, The Chinese University of Hong Kong (Shenzhen), Liu Zhixin, CTO of Wujie Technology, and former chief scientist of artificial intelligence at SF Express, and a food delivery platform. former core technicians.
What technologies are mainly involved in the intelligent distribution system? How to realize the distribution of orders and contact merchants, delivery personnel and consumers?
Wang Zizhuo, co-founder of Shanshu Technology:
It mainly involves two major technologies of machine learning and operational research optimization. The machine learning algorithm is used to predict the delivery time and distance of each order, and the possible response of consumers after timeout; the optimization algorithm provides an optimized distribution plan after the order is received, based on the goals of fast average time and short delivery distance of riders .
Liu Zhixin, CTO of Wujie Technology:
Intelligent distribution tasks include elements such as time and space prediction, order distribution, and route planning, and mainly involve statistics, machine learning, and operational optimization. Takeaway orders have obvious peaks and valleys and a certain degree of randomness, which is mainly reflected in the random order time and the random spatial location of merchants and consumers. Another feature of it is that the timeliness requirements are very fast, and dynamic scheduling is required to allow the delivery boy to receive as many orders as possible and complete the delivery work efficiently.
Former core technicians of the food delivery platform:
The intelligent delivery system mainly involves deep learning, big data, machine learning and duration estimation technology. The core goal of the intelligent scheduling system is to improve the efficiency of the logistics operation of the food delivery platform, in which efficiency and fairness are the two pillars of order dispatching. The intelligent scheduling system learns the delivery data of the riders, defines the riders' grades, and steps up the target orders of riders at all levels, so as to make a capability portrait for each rider and assign the waybills to the most suitable riders.
During the noon and evening peak hours of delivery, the system will take the efficiency of the waybill as the first criterion, and give priority to dispatching orders to high-level riders during the peak period to improve delivery efficiency. The data shows that the system can process up to 80 orders per second at its peak. During the off-peak period of food delivery, the system will emphasize fairness while considering efficiency. Through big data analysis, the order volume of riders is balanced to ensure that the amount of waybills allocated by riders of the same level and team is roughly the same within a certain time span.
In addition to balancing the order quantity of riders, in order to avoid uneven workload of riders, the system will also balance the types of waybills, so that the proportion of long orders and short orders, easy delivery orders and difficult delivery orders in each rider's waybills is approximately it's the same.
2. What principles do intelligent distribution systems usually follow in their work? What is the priority? How are these principles determined?
Liu Zhixin, CTO of Wujie Technology:
This is formulated according to the specific problem. For example, we hope that the per capita efficiency is high, or the service is good, or the cost is the lowest. There may be some factors as constraints of the model, or it may be a multi-objective optimization problem. These principles or mission objectives can be modeled and optimized according to different scenarios and different problems.
As a planner, some further considerations can be reflected in the modeling process. Taking service timeliness as an example, can some elastic margin be added to the timeliness requirements? Or tweak some weights or parameters? From a technical point of view, there is a certain amount of space to achieve.
Wang Zizhuo, co-founder of Shanshu Technology:
For express companies, the satisfaction rate of timeliness is the most important indicator, even prior to cost and profit. In this case, when designing a dispatch plan, the decision-making solution provider will first ensure timeliness, and on this basis help reduce costs and increase revenue. But there are also other factors that companies consider, such as pursuing goals such as revenue, profit, and customer satisfaction. When working with Didi, I found that driver satisfaction is also important.
Former core technicians of the food delivery platform:
The principle is that the global efficiency is optimal. Efficiency means a trade-off between delivery time and cost. This trade-off is related to the stage of operation. In new areas, it is generally preferred to ensure the quality of service. For mature areas, it is necessary to reduce costs on the premise of quality of service.
A core factor for smart scheduling is ETA (Estimate Time of Arrival), which is the time from point A to point B. The source of this time is navigation and the data of the rider's historical movement trajectory. Through the continuous accumulation of data year by year, the algorithm can learn the time it takes for the rider to move between these two points. This time may be achieved by breaking the law, but the system does not perceive it and will think that this target can be achieved.
How does the distribution system optimize the delivery time? Why do takeaway riders experience that the estimated delivery time is shortening year by year? What is the optimization logic behind this?
Wang Zizhuo, co-founder of Shanshu Technology:
The intelligent scheduling system will perform dynamic learning. For example, after completing a day's work, the system will summarize the actual time spent on the order, the final satisfaction rate, the number of customer cancellations or complaints, etc. These data will continue to be fed back to the system to update parameters, so that future optimization can be more accurate.
If the delivery time of the rider is faster, the system will judge that the time required for this journey can be shortened. When dispatching orders in the future, the system may adjust the estimated delivery time. But this depends on the optimization goal in the dispatch. If you blindly aim at the shortest time, some situations mentioned in the article may occur (the average delivery time of the order is getting shorter and shorter).
Liu Zhixin, CTO of Wujie Technology:
Every company does it differently, and I have no way of knowing how other companies do it.
Regarding the estimation of the delivery time, one method is to collect the work data of the delivery boy, combine the GIS information, and re-review the disk to extract features, statistics, or parameters required by the learning model, and then continue to iterate.
But will this method cause some problems behind it, especially these method models inevitably need to rely on data collected in the real world, the real validity of these data is worth thinking about, for example, the system finds that some tasks are completed faster, so adjust The parameters hope that more tasks can be completed according to this efficiency, but the tasks that are completed faster at the beginning are actually biased, and even caused by retrograde behaviors, whether this will bring some misleading to the model. Data is the basis of algorithms, and the authenticity and validity of data is very important. It is worth spending a lot of effort to improve the quality of data collection. Algorithms are not meant to be anti-human.
Former core technicians of the food delivery platform:
Mainly through machine learning, the model with high dispatch efficiency is recorded, and a new algorithm is generated, and the algorithm continuously iteratively optimizes the dispatch logic. The intelligent scheduling system analyzes the restaurant's historical meal delivery data. When the rider receives the order, the system will give priority to the restaurant that delivers the meal faster, allowing the rider to reduce the waiting time at the restaurant.
How does the algorithmic world relate to people? How to consider human factors in algorithm design?
Liu Zhixin, CTO of Wujie Technology:
Algorithms are modeled in the digital world, but there is a gap between the real physical world and the digital world, and there are many uncontrollable variables. Minimizing the divide between the real and digital worlds is a
This is a very important work, including digital twin technology and system, as well as technical personnel and management teams entering the front-line operation to experience the real scene of reality.
Intelligent scheduling is still needed, because it can improve the efficiency of the industry and is helpful to the whole society. In addition, more human factors need to be considered in intelligent dispatching systems. On the one hand, parameters and weights can be adjusted, leaving room for margin. On the other hand, the human behavior against the environment can be used as one of the factors of the model, combined with a large number of planning and simulation, to find a better model expression. For some uncontrollable factors or unexpected factors, it is necessary to combine simulation to see the consequences.
Shanshu Technology CTO Wang Zizhuo:
Algorithms are just a tool. Decision makers hope to achieve a goal through algorithms, and engineers are responsible for finding the best solution to meet the goals. This goal is often not set by the people who do the algorithm, but more by the business department or the management of the enterprise.